Analyzing Experiments
After the experiment is started, it will accumulate users' data and all related metrics.
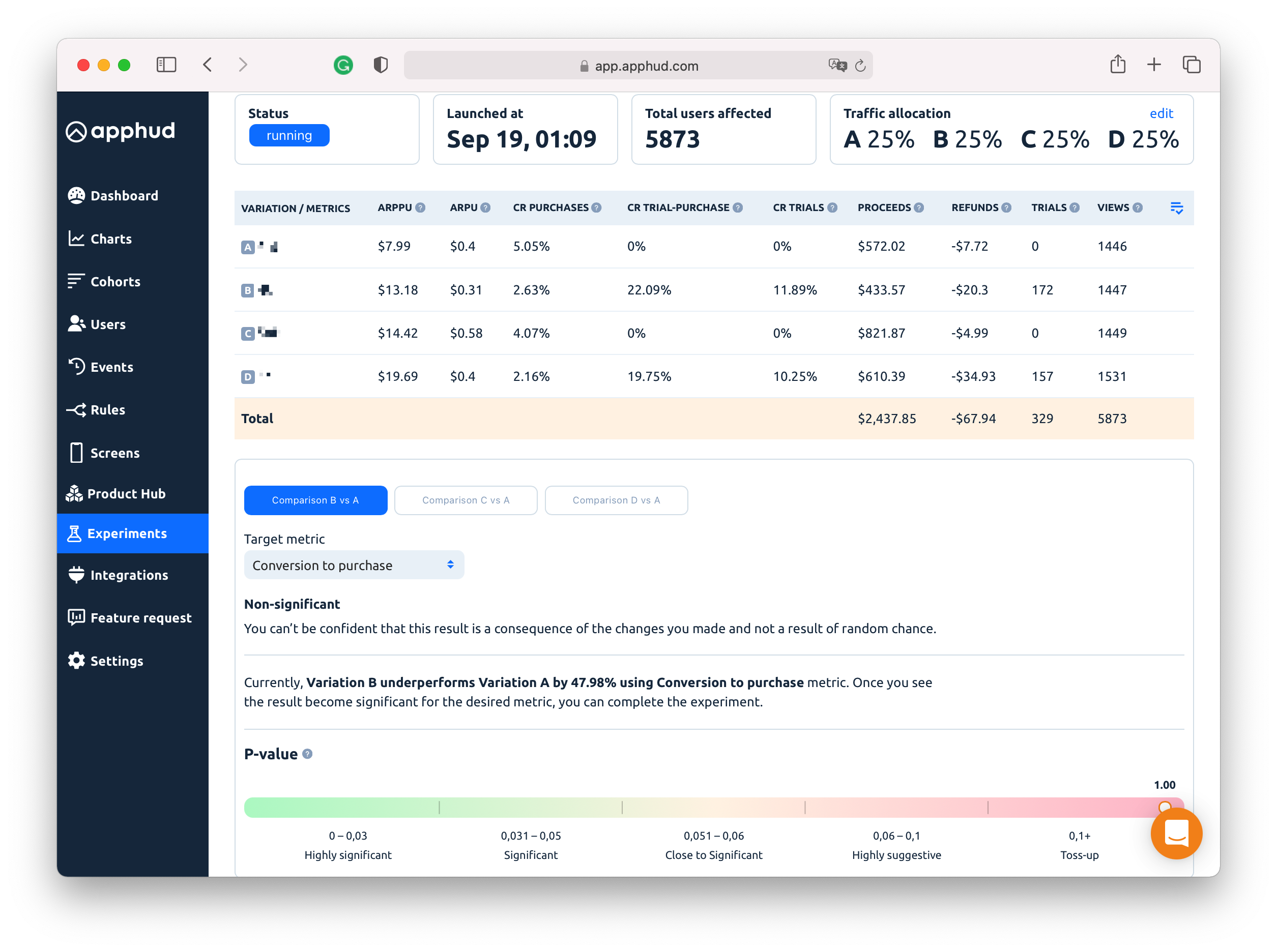
Cohort and Non-Cohort Metrics
Experiment analytics allows to display both cohort and non-cohort metrics.
Cohort metrics are those metrics, for which date picker is applied to a date when users joined the experiment.
Non-cohort metrics are those, for which date picker is applied to an metrics's event date.
Cohort metrics are:
- ARPPU
- ARPU
- ARPAS
- View to Action
- View to Trial
- View to Purchase
- Trial Conversion
- pARPU 1Y
- pARPPU 1Y
- pARPAS 1Y
- pProceeds 1Y
Non-Cohort Metrics are:
Do not compare cohort and non-cohort metricsThis is analytics error to compare cohort metrics with non-cohort. For example, View to Trial metric will not match with the result of dividing Trials by Unique Views, since View to Trial metric allows trials to be after the selected dates.
Target Metrics
View to Purchase
Understand which variation is better in terms of View to Purchase metric.
View to Trial
Understand which variation is better in terms of View to Trial metric.
View to Action
Understand which variation is better in terms of View to Action metric.
ARPU
Understand which variation is better in terms of ARPU metric.
ARPPU
Understand which variation is better in terms of ARPPU metric.
ARPAS
Understand which variation is better in terms of ARPAS metric.
Effect
The "Effect" metric quantifies the relative change between a selected metric in Variation B compared to Variation A. This measurement is expressed as a percentage, indicating how much one variation outperforms or underperforms compared to the other.
Example: If the purchase conversion rate in Variation A is 5% and in Variation B it is 10%, the Effect is +100%. This means Variation B's performance is double that of Variation A, or a 100% improvement.
P-value
The P-value is a statistical metric ranging from 0 to 1, utilized to test hypotheses in experiments. It helps determine whether the observed results are due to chance or are statistically significant.
In our experiments, we set a significance threshold of 5% (P-value = 0.05). A result is considered statistically significant, and thus allows for the rejection of the null hypothesis, if its P-value is less than or equal to this threshold (P-value ≤ 0.05).
We will notify you when the test results are confirmed to be significant based on the P-value criterion.
Experiment Predictions
Experiment Predictions are available if LTV Predictions feature has been added to your app. For more information follow Experiment Predictions guide.
Updated 18 days ago